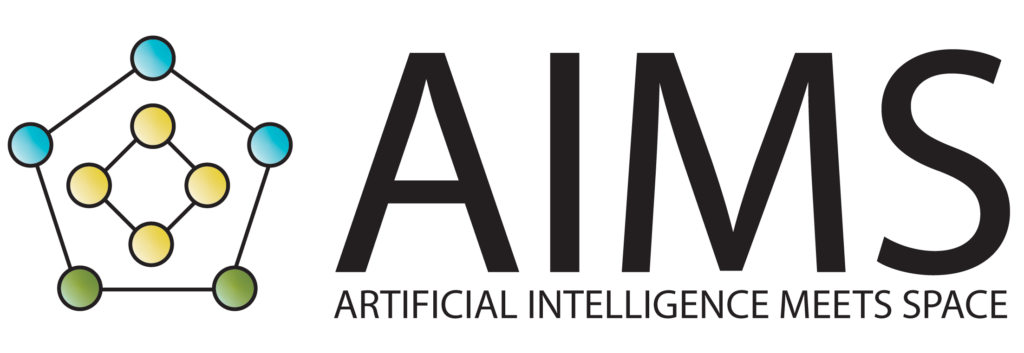
In Collaboration With
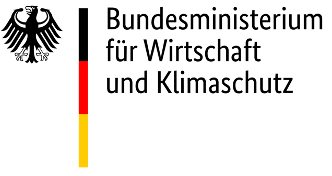
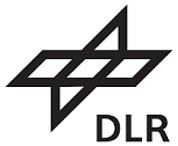
What We Do
AI Meets Space
Integrating AI and Machine Learning with Space Experiments on the ISS
Physics
Two physical projects where the technology is merged with AI to significantly improve image analysis.
Life Sciences
Three life sciences projects utilising AI with data sets to create and enhance predictive and analytical models.
Machine Learning
Four projects centred around machine learning and artificial intelligence to formulate and refine analytical algorithms
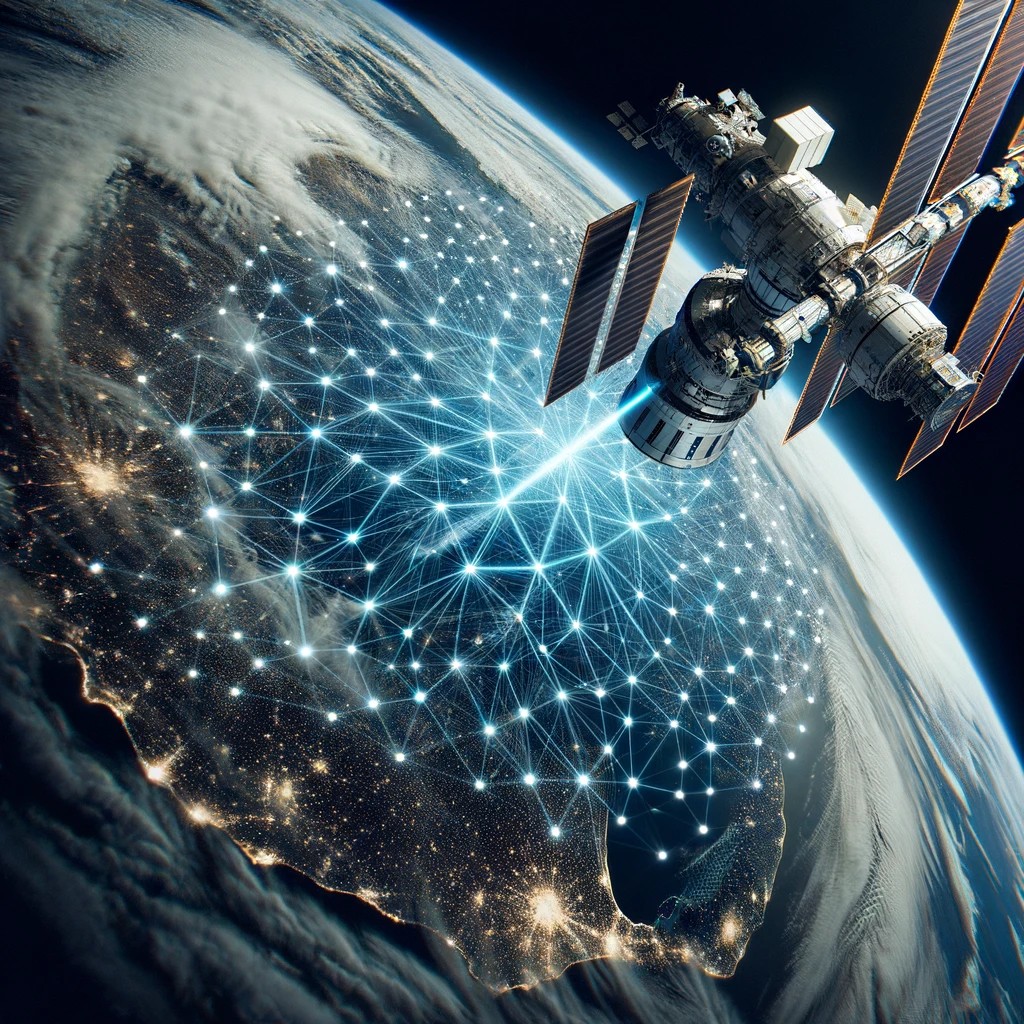
AIMS – Artificial Intelligence Meets Space
The AI expertise network AIMS contributes to further optimizing the scientific use of microgravity platforms such as the ISS, parabolic flights, the drop tower, TEXUS missions or future unmanned platforms as international research platforms, as well as operating and evaluating µg experiments more efficiently. The portability of the developed AI methods should be the focus of the project from the outset. For this reason, the AI methods will be developed and used on an interdisciplinary basis.
The establishment of the AIMS expertise network continues DLR’s current AI efforts in terms of content and contributes to a more efficient use of the ISS, existing µg platforms and future unmanned platforms. Specifically, the use of artificial intelligence will focus on the evaluation of complex, transient, multidimensional data and the autonomous control of experiments. The open software interface and transparent documentation allow future users to quickly and easily adapt the developed methods to their needs. AIMS is creating an AI ecosystem for innovation, which networks players across sectors in order to strengthen the attractiveness of Germany and Europe as a research and business location.
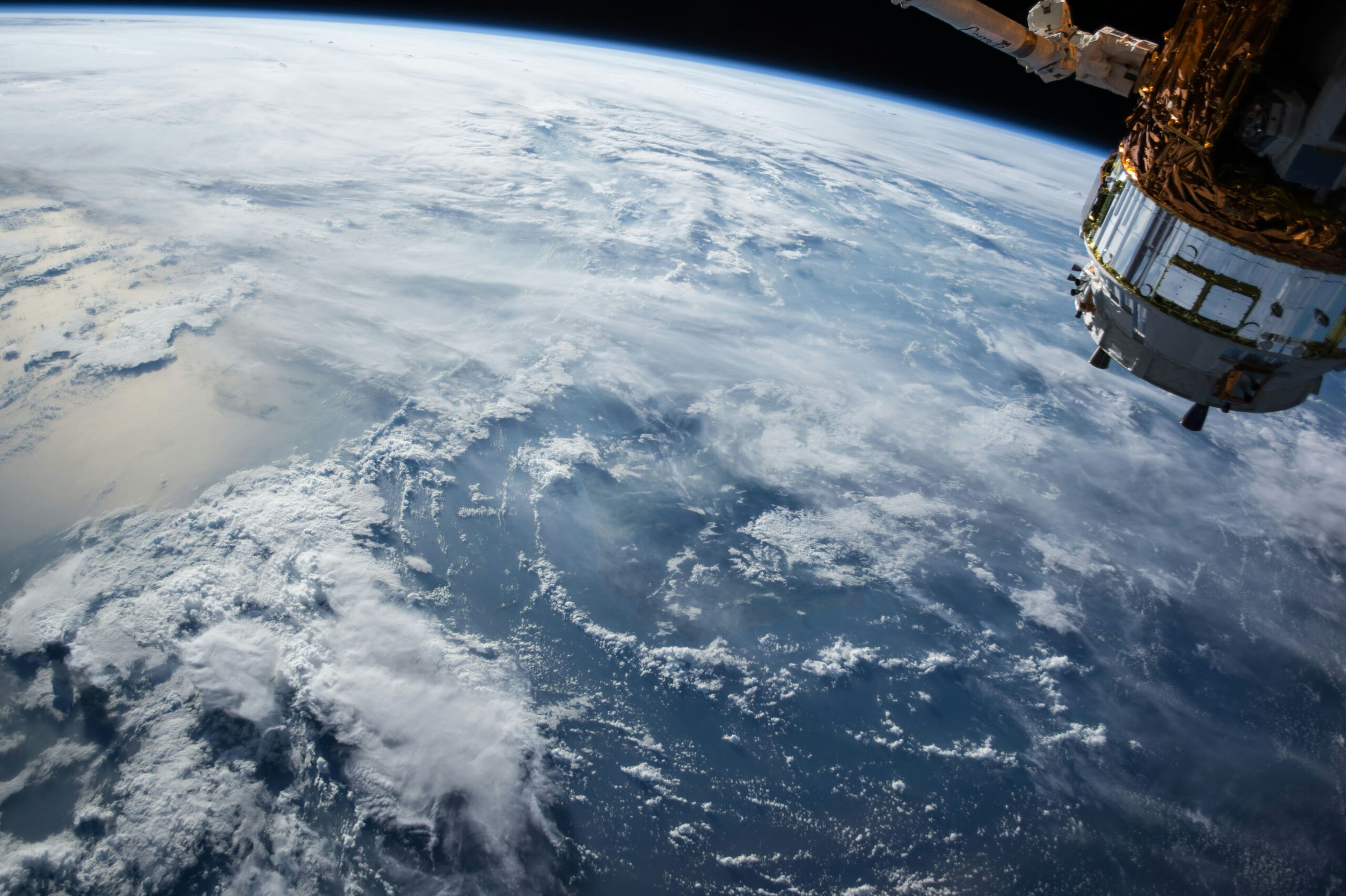
Our Goals
Our Projects
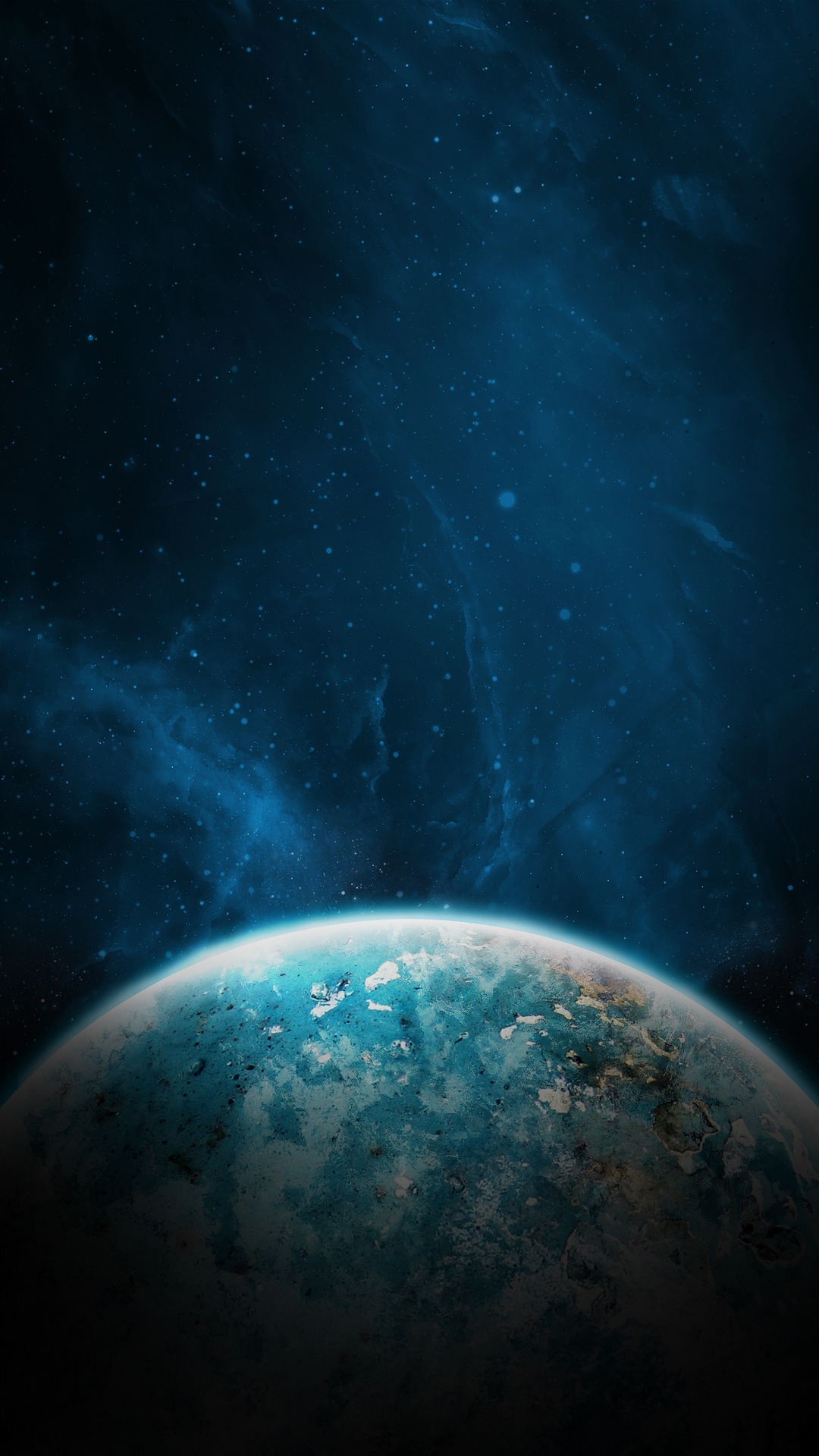
Physics
In the planned mathematical-physical collaborations, the focus is on image processing and image analysis. This can be divided into two different application profiles, (3D) motion analysis and (3D) structure analysis.
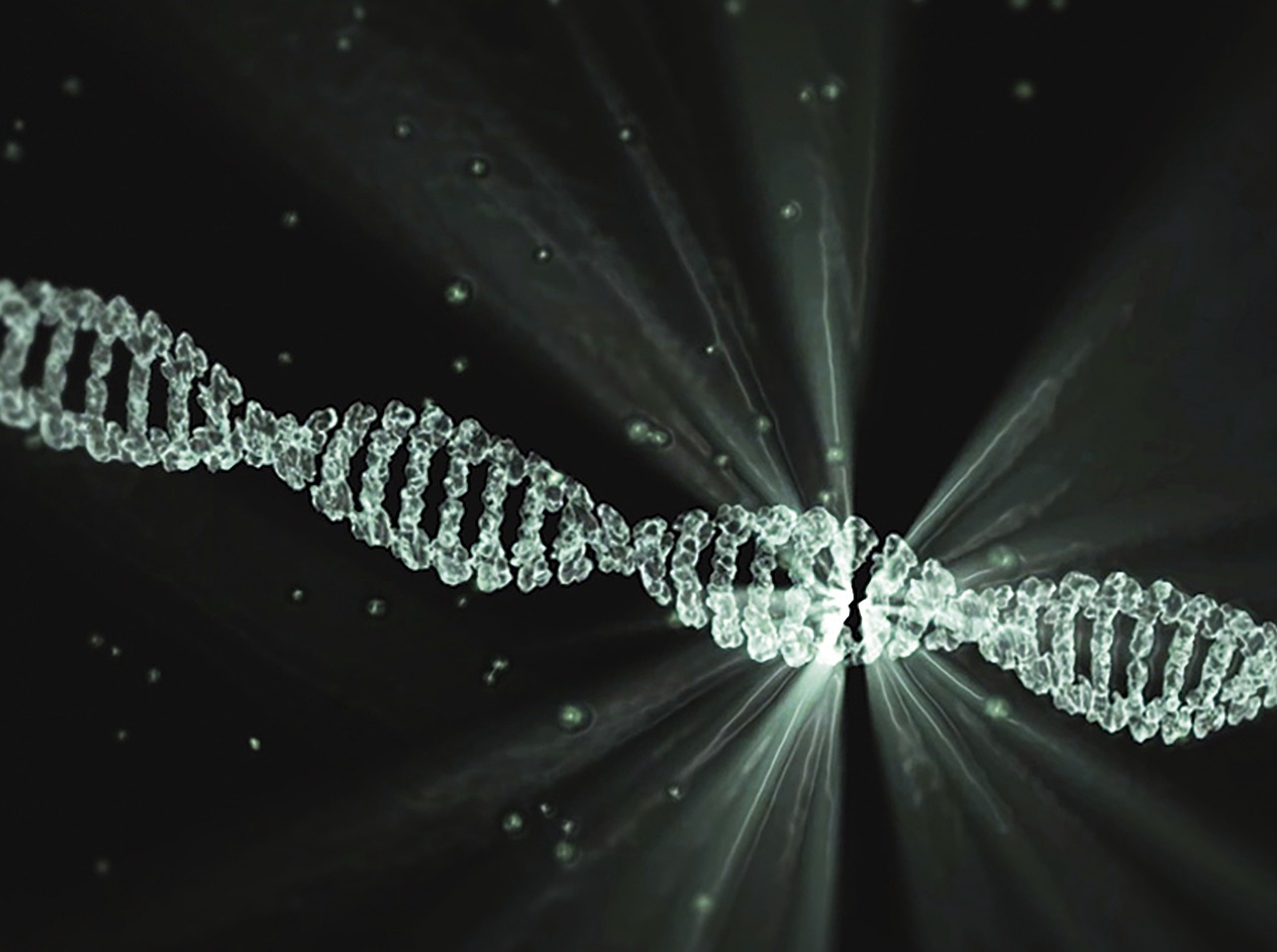
Life Sciences
Data sets generated by experimental groups:
- Transcriptomics expression and proteomics abundance datasets under different conditions to build longitudinal resolved XAI models
- 2D and 3D microscopic images to reduce batch effects and noise as a classification decision leading to Clever Hans predictors
- Creation of XAI models on heterogeneous datasets to extract co-regulatory mechanisms
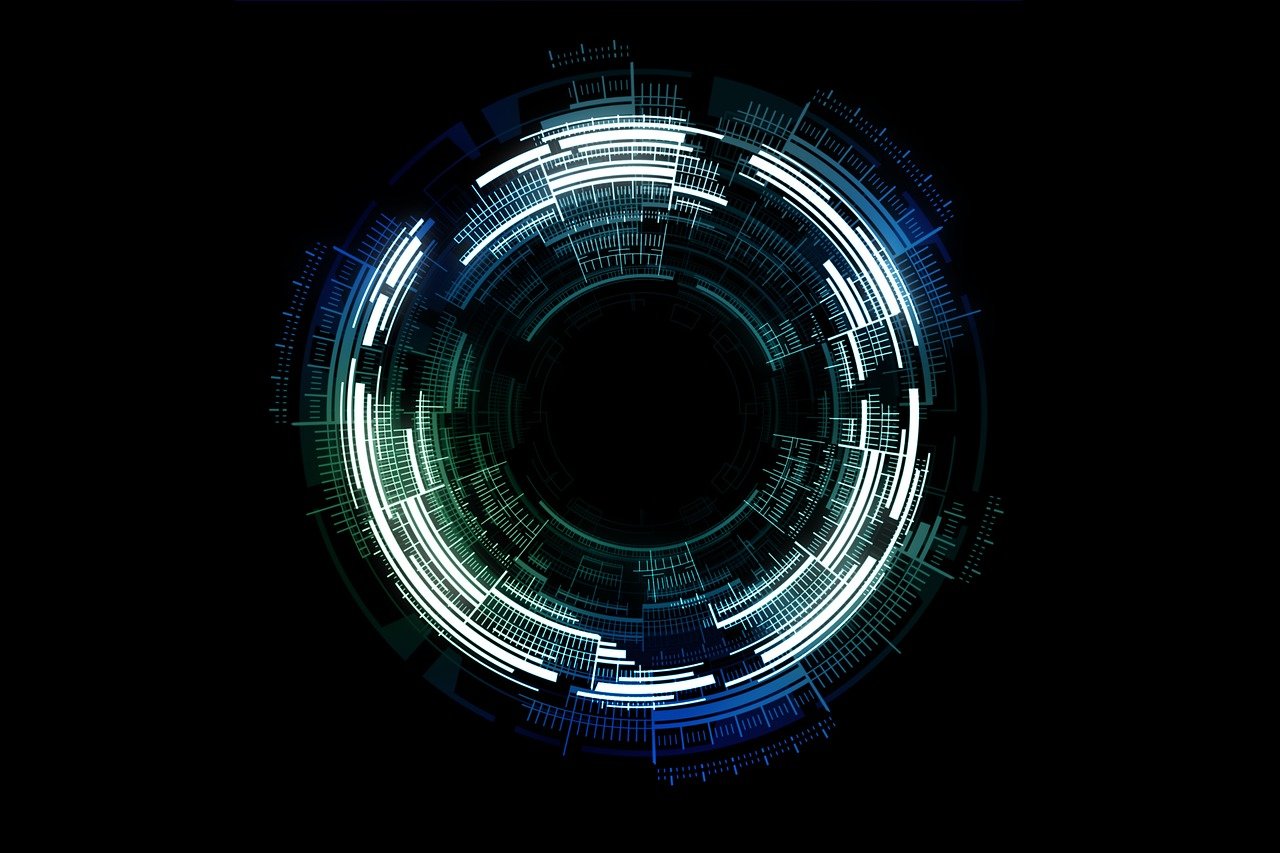
Machine Learning
The AI methods are aimed at control, automation, data generation, optimization, pre-processing, prioritization, reduction and post-processing.
The automation of experiments helps to save crew time and to be able to operate experiments fully autonomously on future unmanned platforms. Another goal is data reduction through prioritization. This should enable experiments to be monitored in real time and avoid longer download times.
The AIMS Ecosystem
Our Partners

News
-
AIMS uses AI-Generated Images on their Website!
Welcome to the AIMS webpage! We hope this is your…
Send Us A Message
Whether you are curious about the our work or want to collaborate with us, reach out to us. We are listening.