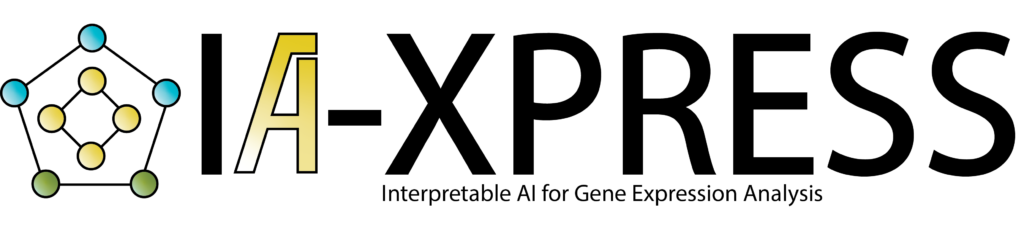
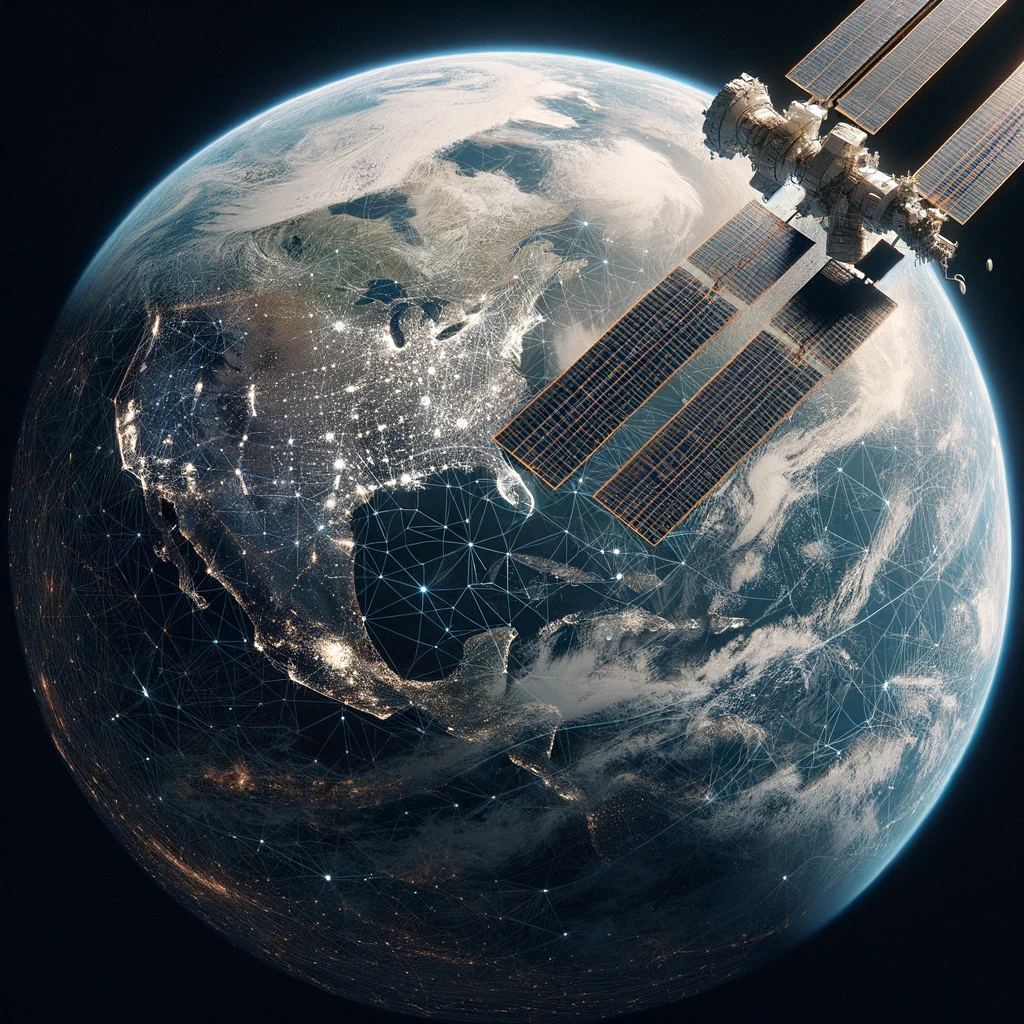
More About IAI-XPRESS
Milestones:
1) Adaptation of the reference vectors to representatives of the data
2) Provision of a smart AI for the classification of gene expression data
IAI-XPress – Interpretable Artificial Intelligence for gene expression data is part of AIMS, tackling the challenge of applying AI methods to scarce but high-dimensional data. A major challenge is that the amount of experimental data available for an observing task, especially one involving rockets or zero-g, is small compared to the very large number of variables to be measured. Simply put, we have far more variables to consider than actual data points to work with. This poses a significant problem for traditional deep learning models. One problem with these models is that they often become overly specialized to the noise in the training data. This makes them unable to learn meaningful patterns.
Our objective is to innovate a smart AI model that can learn from this limited data while remaining compact. A promising solution lies in the development of knowledge-informed and interpretable models, which leverage the wealth of existing expertise in biology to guide the modeling process. By tapping into established databases and incorporating expert knowledge into the model design, we can create AI systems that are not only highly accurate but also more transparent, inherently explainable, and based on a deep understanding of the underlying biological mechanisms. This approach enables us to distill complex relationships between genes and their expression levels into interpretable models, allowing researchers to gain insights into the discrimination and make the decision process transparent. The result is the so-called Biological Informed Generalized Matrix Learning Vector Quantization Model (BI-GMLVQ).
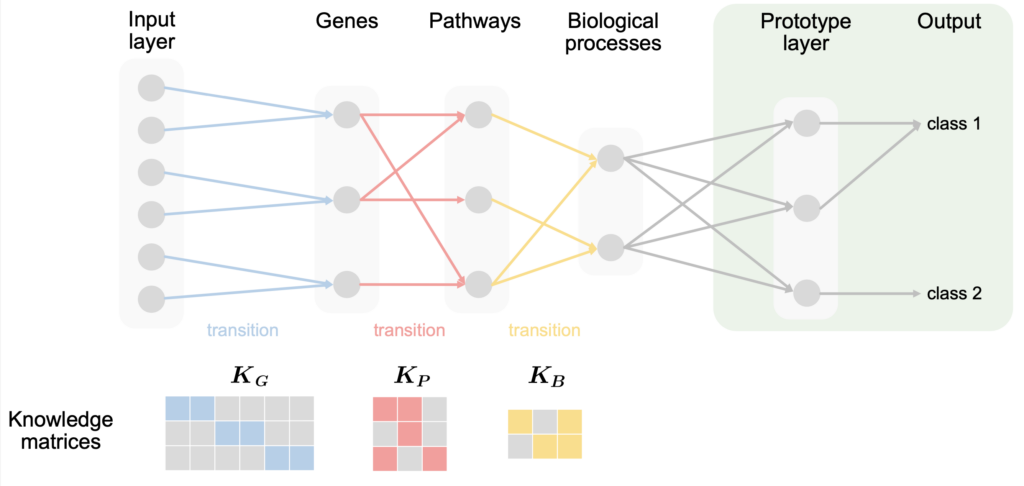
The rough structure of the model is A more detailed description of the model and the method can be found in:
Voigt, J., Saralajew, S., Kaden, M., Bohnsack, K. S., Reuss, L. V., and Villmann, T. (2024). Biologically-Informed Shallow Classification Learning Integrating Pathway Knowledge. In BIOSTEC (1) (pp. 357-367).
For further publications, which were created in the projects, can be found here (Link: https://vivo.hs-mittweida.de/vivo/display/personidm24124)
We collaborate closely with the team from Xtras (link to https://aims.science/xtras/).
Our Team
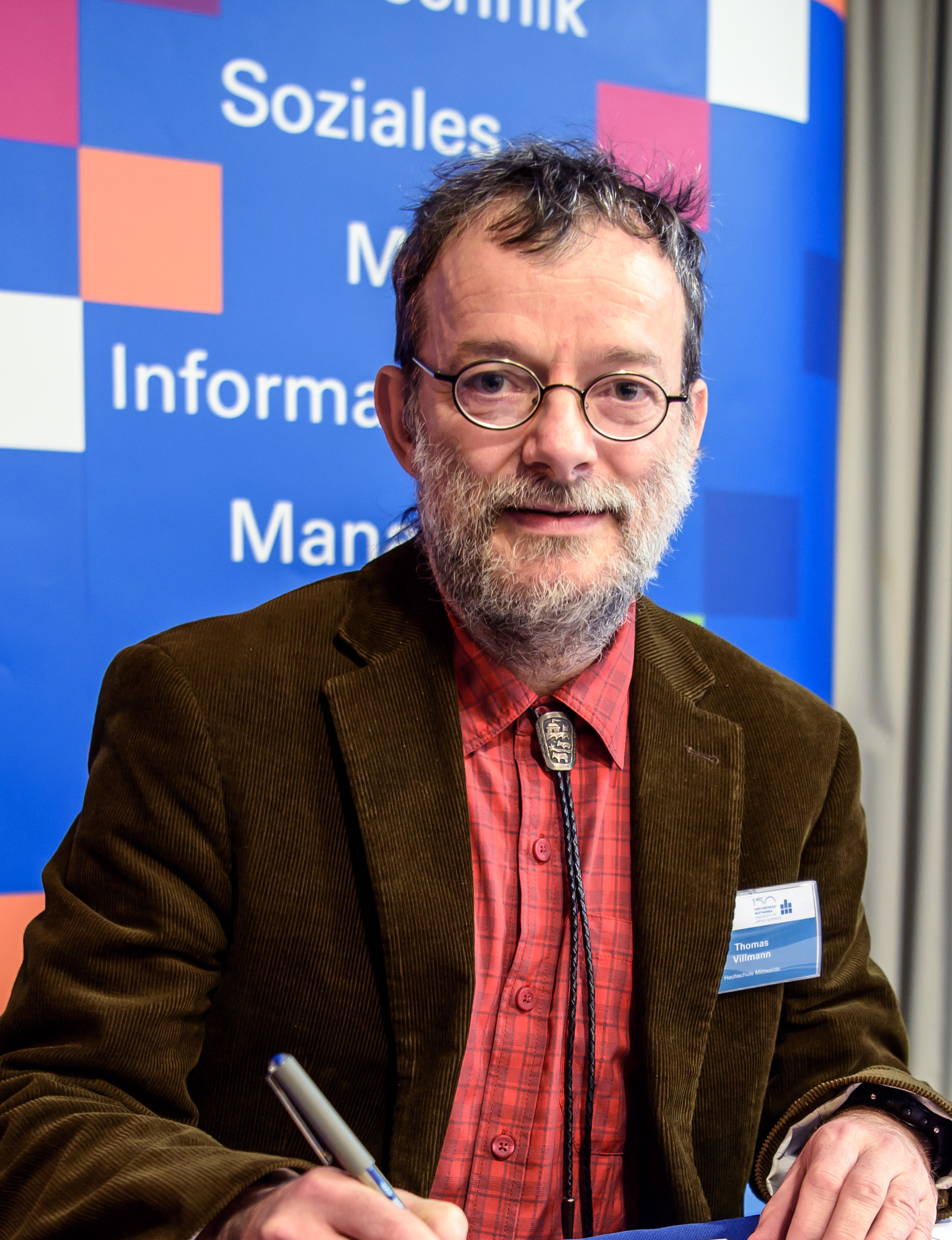
Prof. Thomas Villman
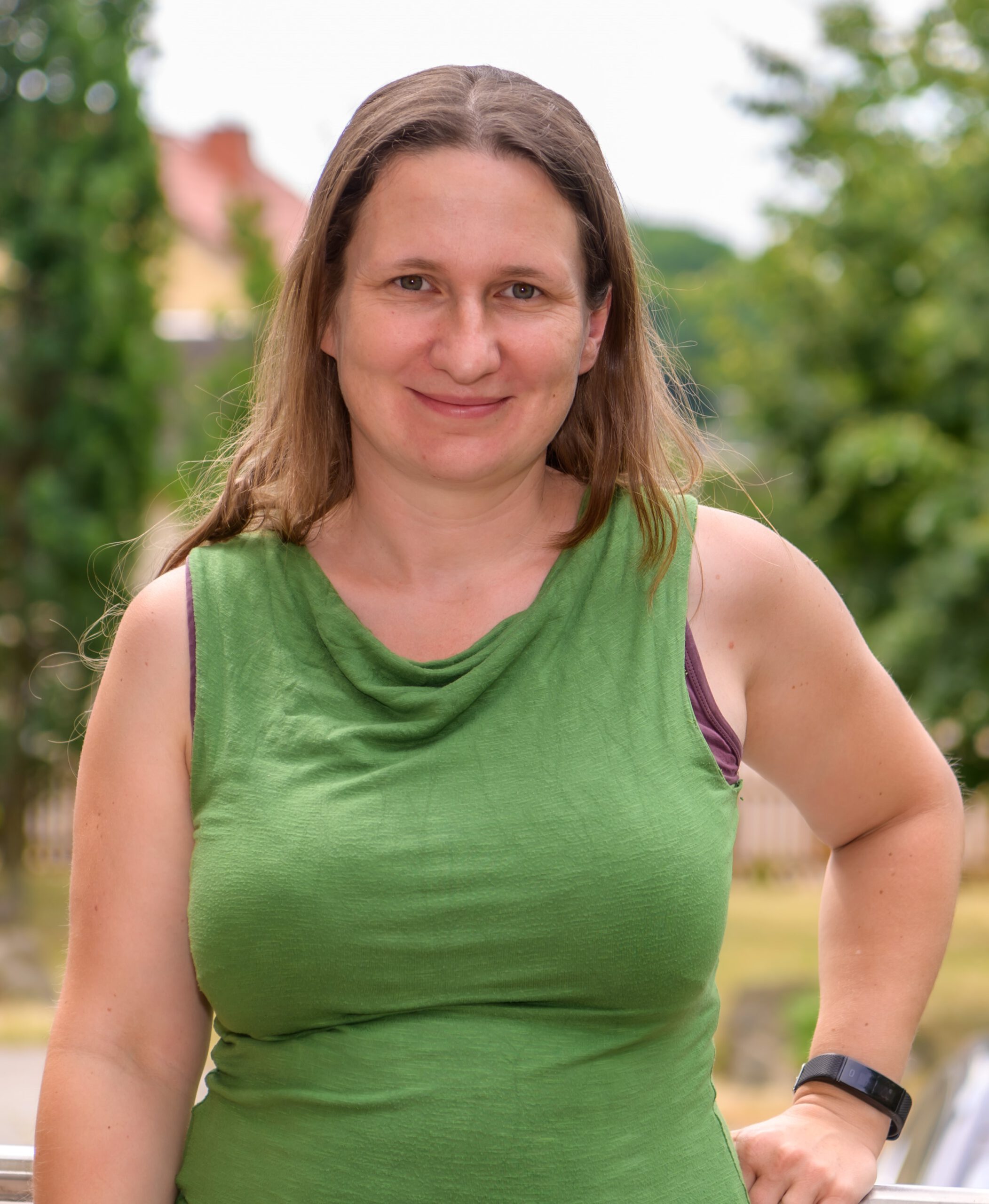
Dr. Marika Kaden
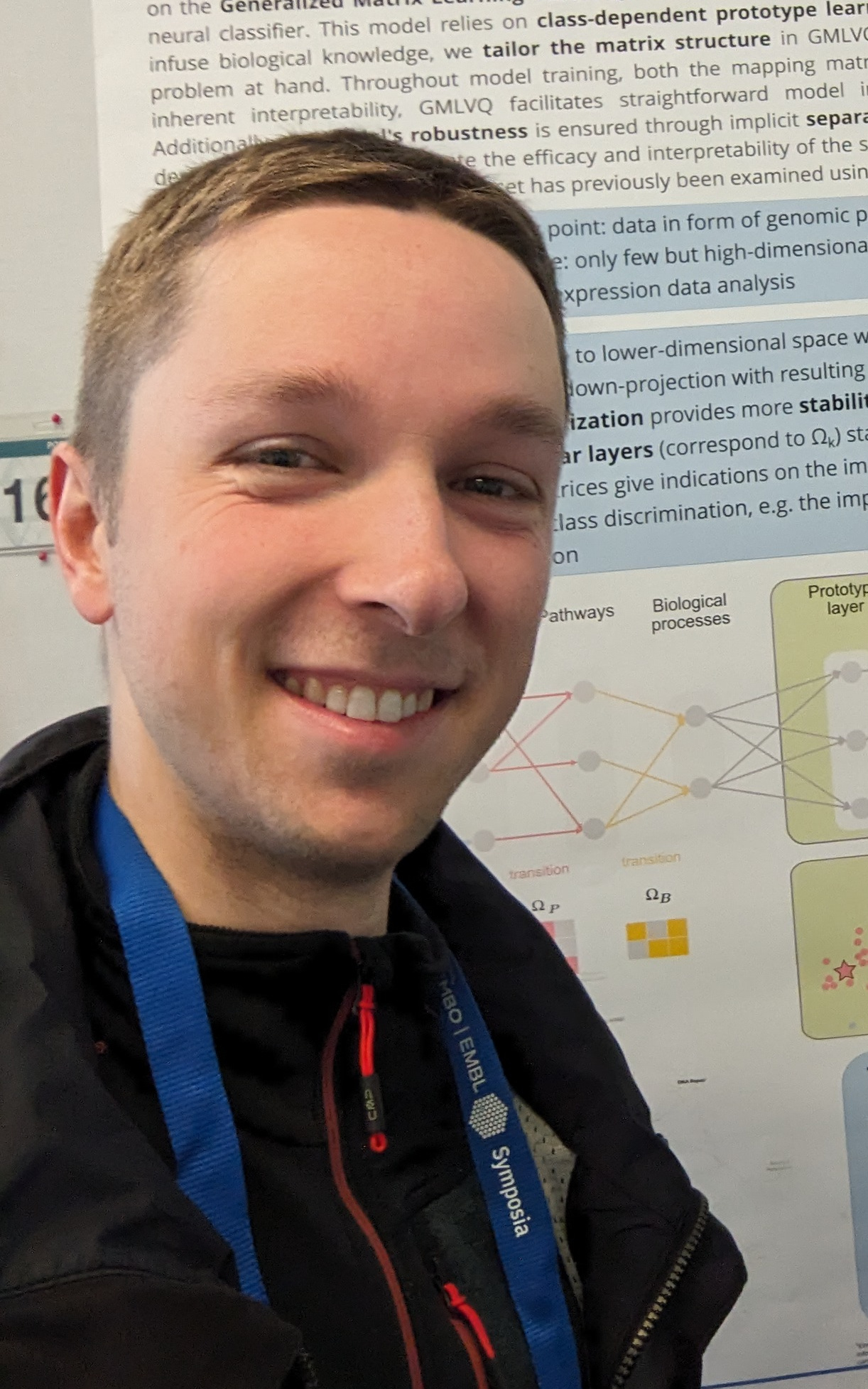
Julius Voigt
Send Us A Message
Whether you are curious about the our work or want to collaborate with us, reach out to us. We are listening.